16. Hough Transform
Hough Transform Quiz
Question:
Now it's your turn to play with the Hough Transform on an edge-detected image. You'll start with the image on the left below. If you "Test Run" the quiz, you'll get output that looks like the center image. Your job is to modify the parameters for the Hough Transform and impose a region of interest mask to get output that looks like the image on the right. In the code, I've given you a framework for defining a quadrilateral region of interest mask.
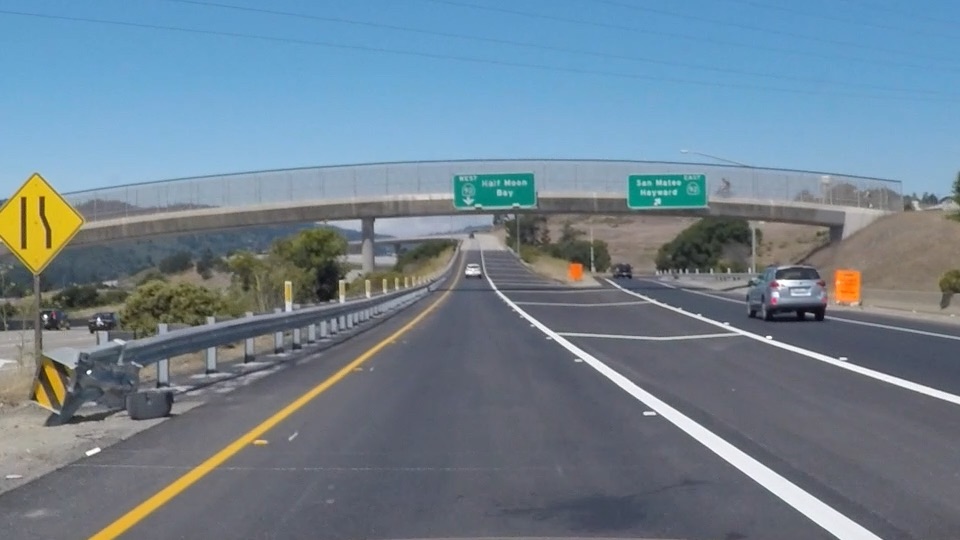
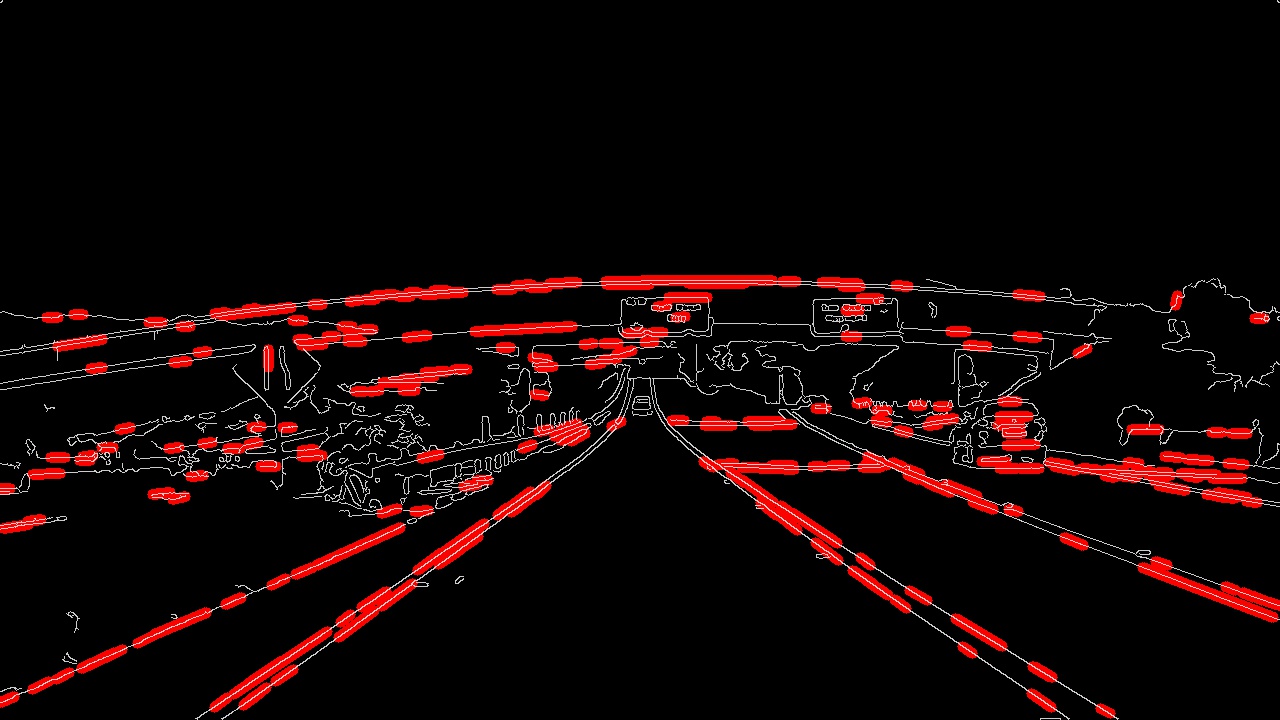
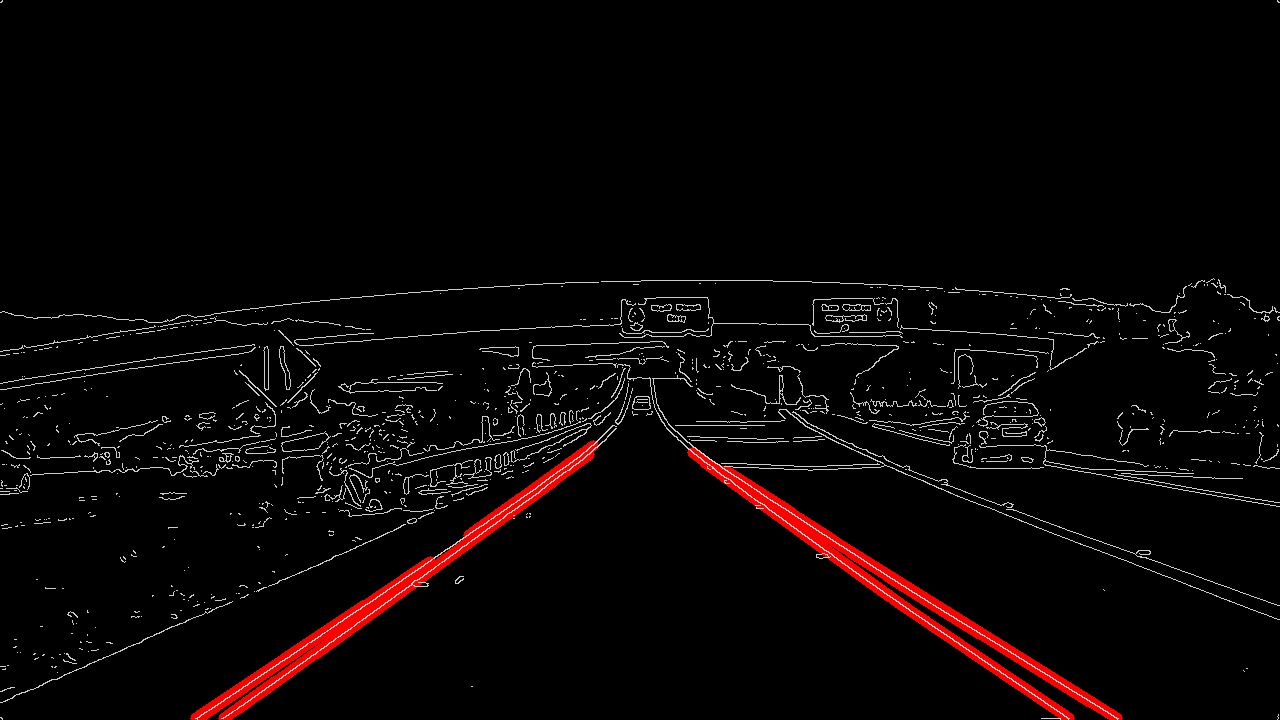
Start Quiz:
import matplotlib.pyplot as plt
import matplotlib.image as mpimg
import numpy as np
import cv2
# Read in and grayscale the image
image = mpimg.imread('exit-ramp.jpg')
gray = cv2.cvtColor(image,cv2.COLOR_RGB2GRAY)
# Define a kernel size and apply Gaussian smoothing
kernel_size = 5
blur_gray = cv2.GaussianBlur(gray,(kernel_size, kernel_size),0)
# Define our parameters for Canny and apply
low_threshold = 50
high_threshold = 150
edges = cv2.Canny(blur_gray, low_threshold, high_threshold)
# Next we'll create a masked edges image using cv2.fillPoly()
mask = np.zeros_like(edges)
ignore_mask_color = 255
# This time we are defining a four sided polygon to mask
imshape = image.shape
vertices = np.array([[(0,imshape[0]),(0, 0), (imshape[1], 0), (imshape[1],imshape[0])]], dtype=np.int32)
cv2.fillPoly(mask, vertices, ignore_mask_color)
masked_edges = cv2.bitwise_and(edges, mask)
# Define the Hough transform parameters
# Make a blank the same size as our image to draw on
rho = 1 # distance resolution in pixels of the Hough grid
theta = np.pi/180 # angular resolution in radians of the Hough grid
threshold = 1 # minimum number of votes (intersections in Hough grid cell)
min_line_length = 5 #minimum number of pixels making up a line
max_line_gap = 1 # maximum gap in pixels between connectable line segments
line_image = np.copy(image)*0 # creating a blank to draw lines on
# Run Hough on edge detected image
# Output "lines" is an array containing endpoints of detected line segments
lines = cv2.HoughLinesP(masked_edges, rho, theta, threshold, np.array([]),
min_line_length, max_line_gap)
# Iterate over the output "lines" and draw lines on a blank image
for line in lines:
for x1,y1,x2,y2 in line:
cv2.line(line_image,(x1,y1),(x2,y2),(255,0,0),10)
# Create a "color" binary image to combine with line image
color_edges = np.dstack((edges, edges, edges))
# Draw the lines on the edge image
lines_edges = cv2.addWeighted(color_edges, 0.8, line_image, 1, 0)
plt.imshow(lines_edges)
Solution:
Here's how I did it: I went with a
low_threshold
of 50 and
high_threshold
of 150 for Canny edge detection.
For region selection, I defined
vertices = np.array([[(0,imshape[0]),(450, 290), (490, 290), (imshape[1],imshape[0])]], dtype=np.int32)
I chose parameters for my Hough space grid to be a
rho
of 2 pixels and
theta
of 1 degree (pi/180 radians). I chose a
threshold
of 15, meaning at least 15 points in image space need to be associated with each line segment. I imposed a
min_line_length
of 40 pixels, and
max_line_gap
of 20 pixels.
With these parameters, I'm picking up the lanes lines and nothing else, so looks like a decent solution!
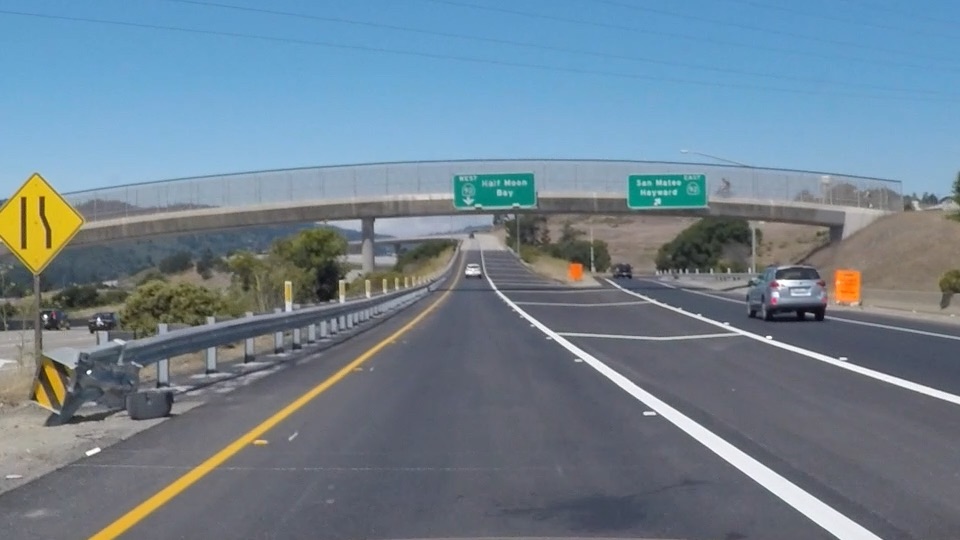
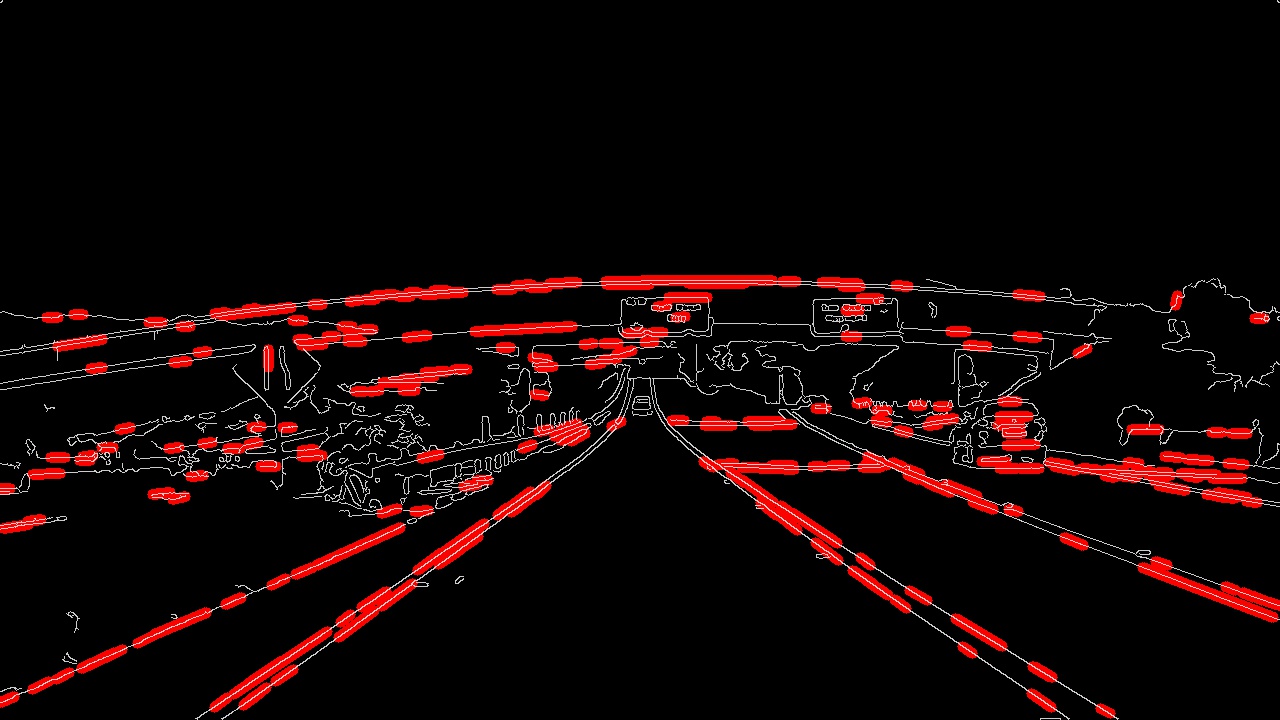